
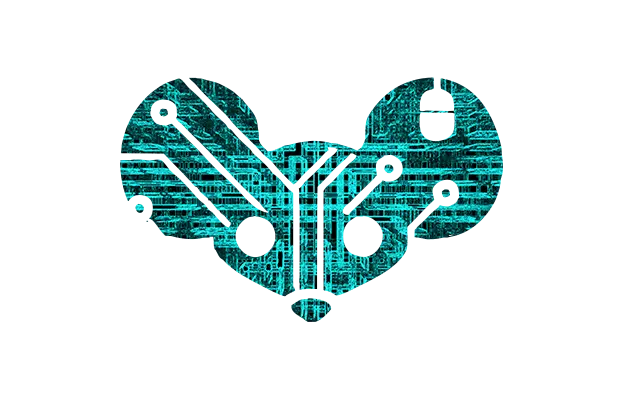
Most “50 MP” cameras are actually quad Bayer sensors (effectively worse resolution) and are usually binned 2x to approx 12 MP.
The lens on your phone likely isn’t sharp enough to capture 50 MP of detail on a small sensor anyway, so the megapixel number ends up being more of a gimmick than anything.
Yeah, you can certainly get it to reproduce some pieces (or fragments) of work exactly but definitely not everything. Even a frontier LLM’s weights are far too small to fully memorize most of their training data.